The ALTEMIS Approach
The ALTEMIS Approach
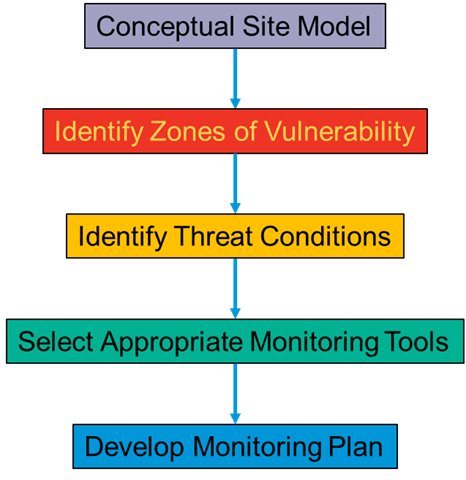
The Savannah River Site F-Area plume is an evolving, complex geochemical system out of equilibrium with baseline conditions. The approach used at F-Area was to:
- Develop a robust conceptual site model and determine the implications of the remedial actions, forming the basis of a long-term monitoring plan.
- Identify zones of vulnerability based on the conceptual site model. Zones of vulnerability are locations where residual contamination is expected to exist in an attenuated form in the future. Threat conditions, which are changes in environmental conditions that could remobilize attenuated contaminants, were identified for each of the zones of vulnerability and some vary between zones.
- Select appropriate monitoring tools by obtaining information with minimal sample collection and analysis, which includes tailoring the tools to the nature of the vulnerability.
- Implement a long-term monitoring plan.
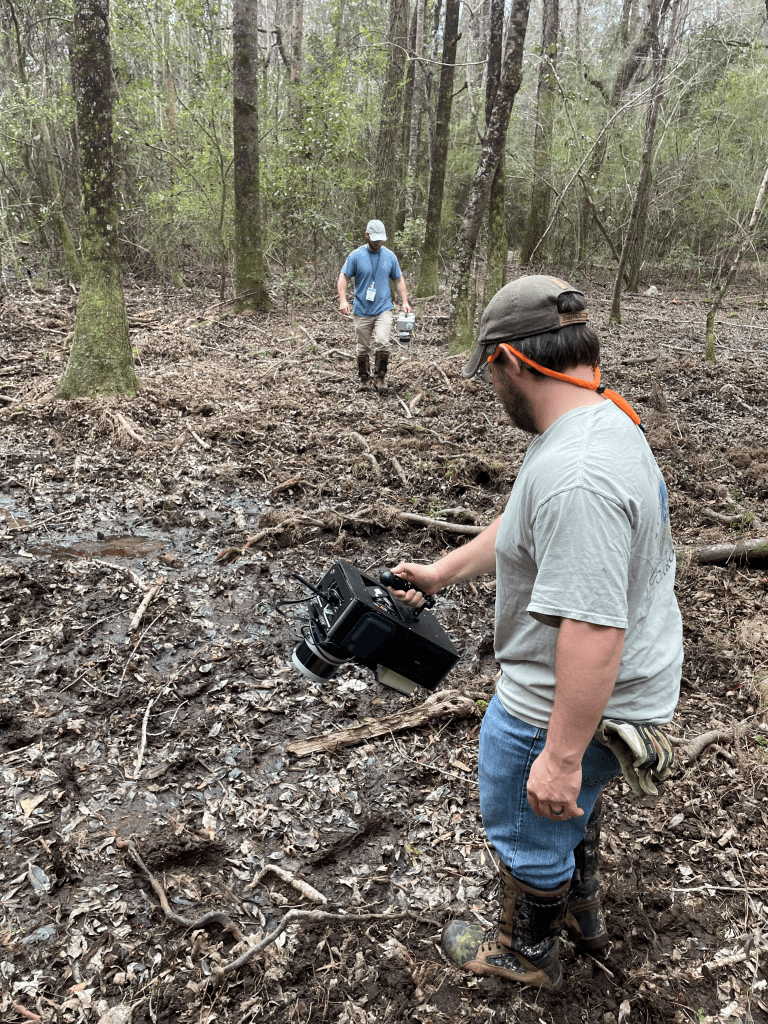
Main Objective: Study how contaminants behave and change long-term.
The primary geochemical concerns for long-term monitoring are conditions that could reverse contaminant attenuation or otherwise increase release of contaminants over decades or even centuries. Historical data and information already acquired include:
- Contaminant identification
- Contaminant measurements and trends
- Master/chemical variables (pH, specific conductivity, etc.)
- Physical forces (water level, evapotranspiration, temperature, etc.)
- Conceptual Site Model
- Identification of zones of vulnerability through the Conceptual Site Model
Installed sensor networks determined longer-term changes to wetland geochemistry. Modeling evaluated likely contaminant behavior in response to longer-term scenarios, such as changes related to extreme rainfall events, changes in the course of the local stream, and changes in vegetation expected to occur in an unmaintained landscape.
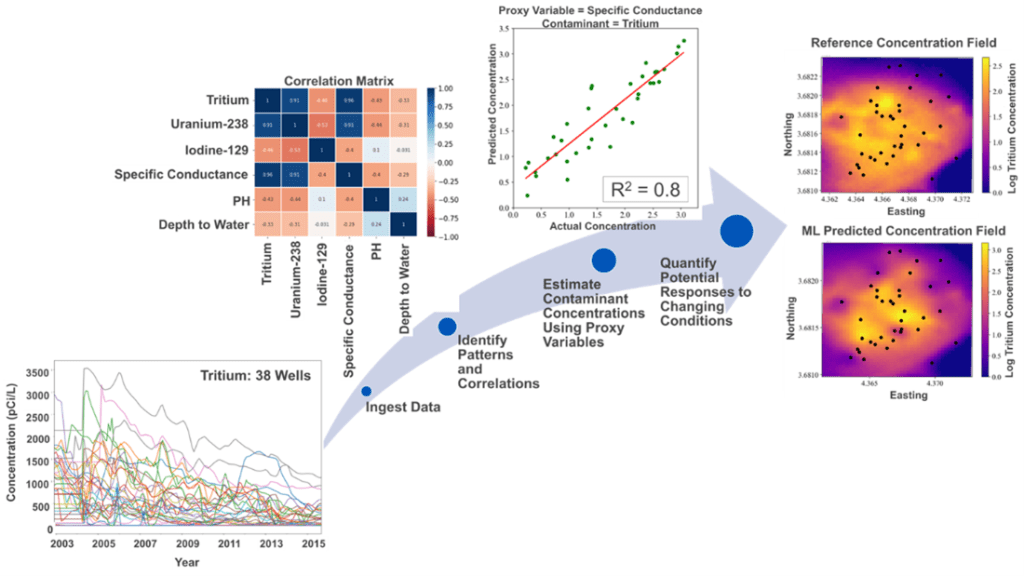
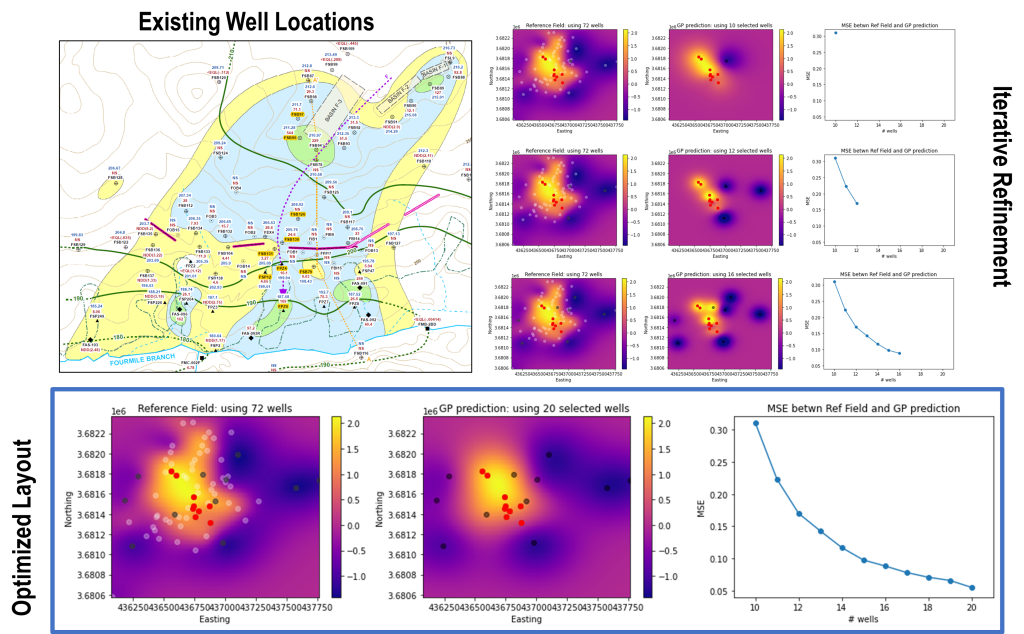
Main Objective: Develop software to perform various machine-learning and data analytical tasks for groundwater data, which could be used across Department of Energy sites.
Monitoring for groundwater contamination is done primarily for compliance purposes, with data often used to flag any regulatory exceedance. Those data are then archived without further analytics.
SRNL developed various tools for machine learning and data analytics for advanced analysis of soil and groundwater data, including unsupervised machine learning for identifying spatiotemporal patterns of contaminant concentrations, or quantifying their responses to climate variables (e.g., flooding or drought).
SRNL also developed supervised machine learning for evaluating the ability to estimate and predict contaminant concentrations, based on measurable parameters in the groundwater, as well as the effectiveness of well configuration, to capture contaminant concentration distributions.
The ALTEMIS team is demonstrating the use of reactive transport modeling in support of monitoring strategies by simulating the impact of climate deviations on residual contaminants and the range of contaminant remobilization and changes in plume direction.
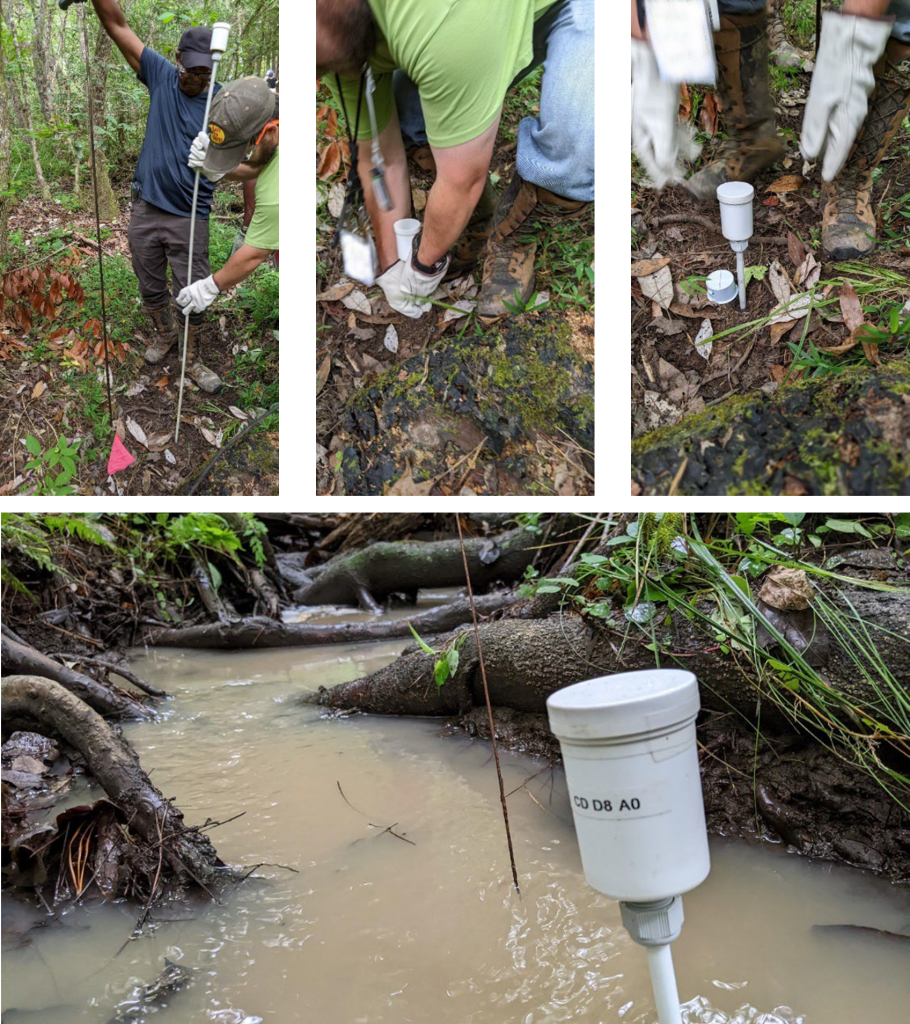
Main Objective: Monitor controlling variables to provide an early-warning system for unanticipated changes in plume behavior.
Real-time sensors are a powerful alternative to conventional groundwater sampling and laboratory analysis. Due to established regulations, these sensors will not eliminate groundwater sampling, however, this strategy reduces the number of wells and frequency of sampling.
The ALTEMIS team completed installation of the sensor package system in Savannah River Site F-Area in 2023. The sensors communicate through automated data transmission and visualization technologies. Instead of waiting for results from quarterly sampling, this integrative system acts as a sentinel for changes in plume dynamics. The combination of various sensors in this integrated network provides researchers with a complete snapshot of plume conditions.
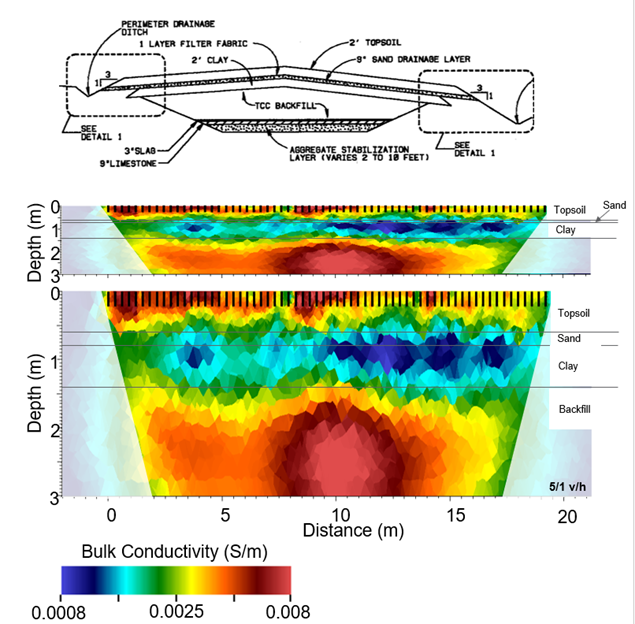
Spatial monitoring methods remotely detect surface and subsurface properties helping to assess behavior and performance of the system. These methods identify significant irregular changes over time providing early warning to researchers in real time. Given the spatial and temporal scales required for long-term monitoring of remediated Department of Energy Environmental Management sites, spatial sensing systems must be autonomous enough to limit costs by reducing human effort. Additionally, they must be robust enough to operate for long periods of time without maintenance in remote areas.
The wetland subsurface is characterized by integrating tools, such as Electromagnetic Induction and Ground Penetrating Radar. These techniques are sensitive to variations in subsurface electrical properties and, therefore, are valuable tools for efficiently estimating soil characteristics. The information obtained can help understand the migration and retention of contaminants in the wetlands.
Combining Ground Penetrating Radar and Electromagnetic Induction measurements along a transect of the surface can provide a 2-D picture of the stratigraphy of the subsurface. Autonomous electrical resistivity and phase tomography monitoring has the potential to achieve rapid and automated detection and identification of contaminant plumes, as well as water infiltration within the vadose zone. In parallel, remote sensing-based characterization can identify subsidence, surface runoff patterns, and infiltration hotspots.
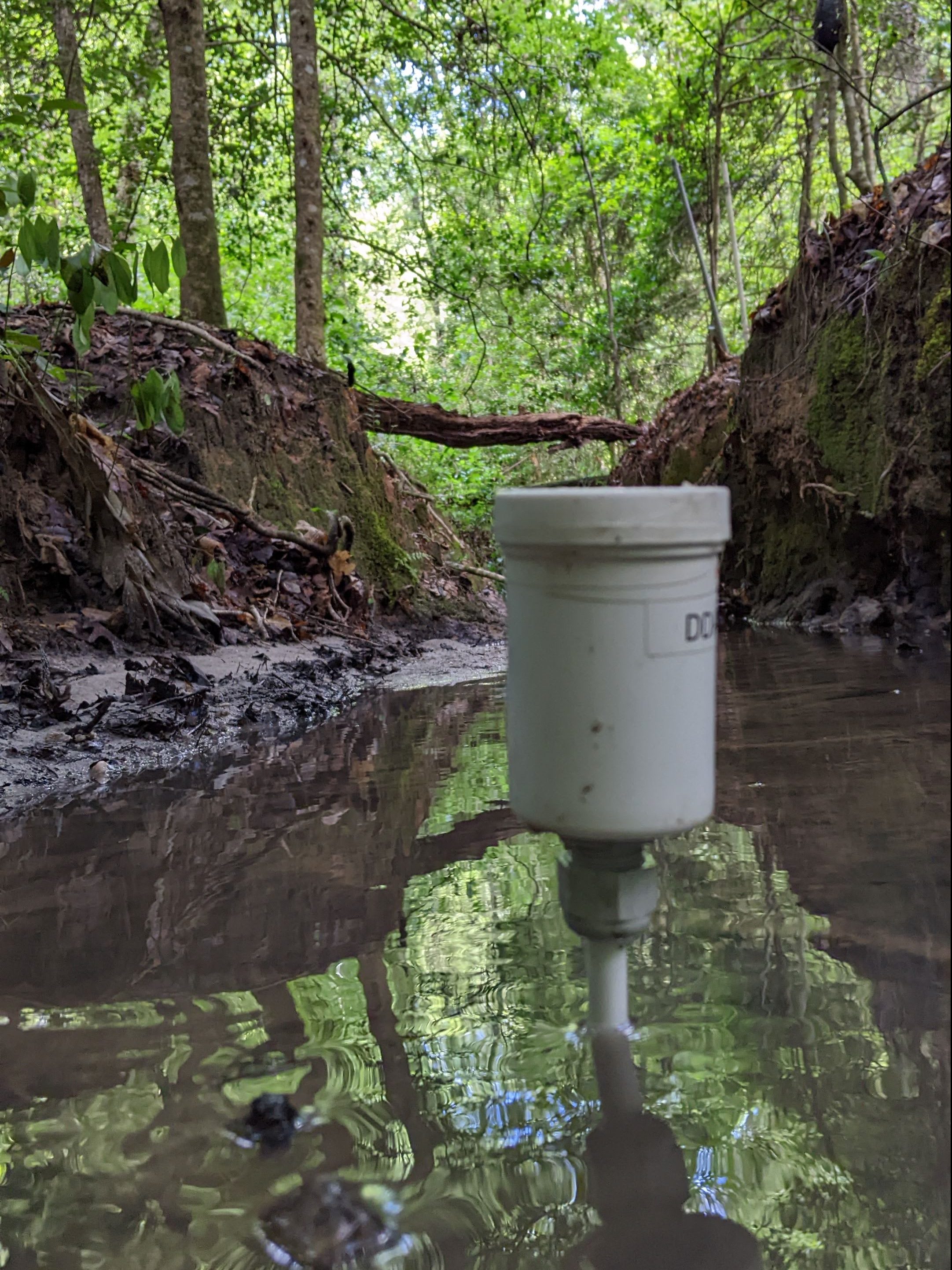
Main Objective: To obtain baseline data and validation of the ALTEMIS approach.
Before this approach can be implemented, evaluation of historical monitoring data needs to occur to identify important parameters used to develop a strategy. This evaluation includes the use of existing groundwater chemistry data for identifying relevant physical forces and chemical variables responsible for the remobilization and attenuation of contaminants.
Data analytics can also be used to establish correlations between contaminants and chemical variables, like pH or redox. This allows the use of chemical variables as surrogates to estimate contaminant concentration. The Artificial Intelligence/Machine Learning approach can optimize monitoring by reducing sampling frequency and number of wells but still capture the spatial distribution of the plume. As the site transitions to long-term monitoring, data from traditional monitoring can be used to compare and validate the efficacy and robustness of the new approach.
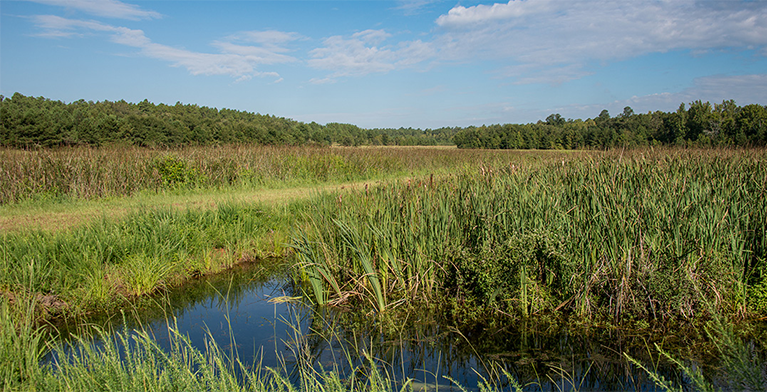
Main Objective: Engage regulators and stakeholders to support the implementation of ALTEMIS.
One of the core objectives of ALTEMIS is to reduce the need for standard monitoring well sampling through applying innovative tools and methods, which are both cost-effective and efficient for long-term implementation.
Until the South Carolina Department of Health and Environmental Control accepts the reduction in frequency of sampling, no changes can be made. Data collected from the sensors are being used to evaluate the innovative versus the baseline monitoring plan. Initial Artificial Intelligence/Machine Learning evaluation of the results show that sensor data from only 25 sensor locations can provide an optimal representation of the Savannah River Site F-Area plume. In addition, sensors can provide sentinel notification for the plume’s behavior and provide real-time notification of any unexpected changes.
Researchers are now able to monitor and respond to changes on a daily basis rather than relying on quarterly sampling. It is important to engage regulators and stakeholders regularly in the proposed strategy so they can understand fully the benefits of ALTEMIS at Savannah River Site F-Area.