Applications & Expansion
Applications & Expansion
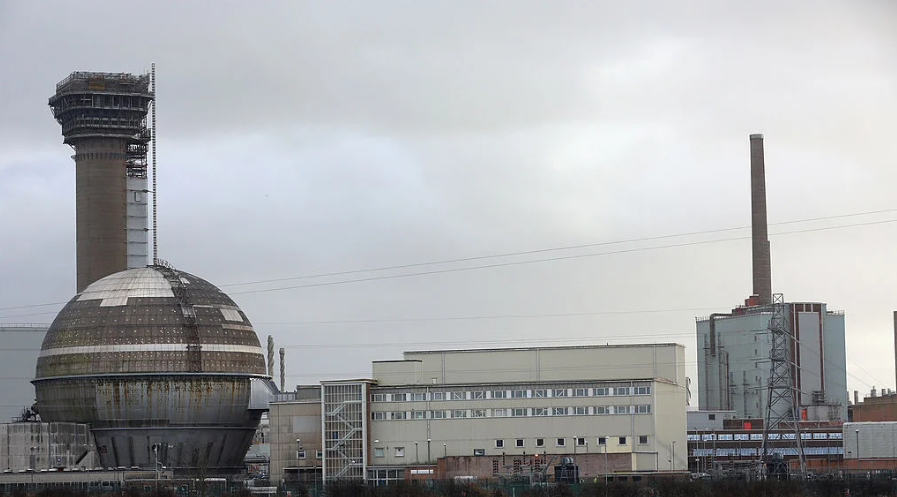
Savannah River National Laboratory is working with Sellafield Ltd. in designing and optimizing a groundwater sensor network for monitoring radionuclide contaminants from underground waste tanks. The groundwater dynamics at the Sellafield Site have the potential for substantially different characteristics than those at the Savannah River Site, given the proximity to the ocean and nearby river. The objectives of the monitoring paradigm are to define the migration of contamination and legacy contamination within the groundwater plume, creating new opportunities for algorithmic development that will be transferrable to additional sites.
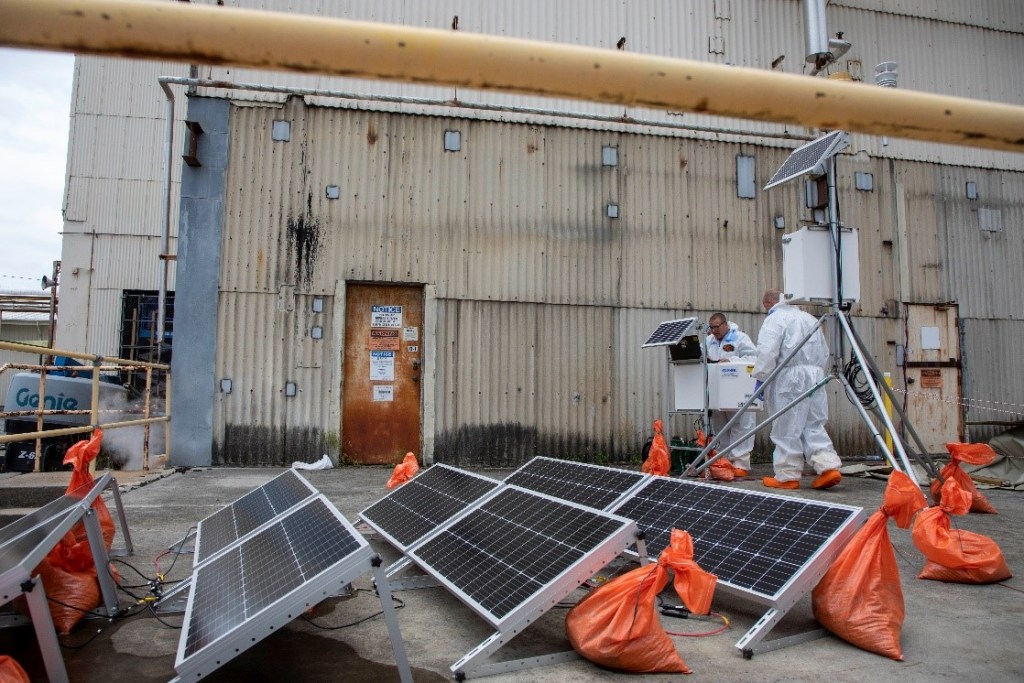
Savannah River National Laboratory has an ongoing effort to explain mechanisms controlling migration of mercury vapors and recurrent mercury concentration changes in legacy-use facilities, which will ultimately contribute to improved worker safety during deactivation and decommissioning operations.
Y-12 installed sensor packages at its legacy-use facility and monitoring is ongoing. SRNL is using Artificial Intelligence/Machine Learning technologies to identify volatile contaminant trends and distinguish the controlling variable(s) resulting in high concentrations in the air. Once identified, these variables can serve as leading indicators of concentration changes and potentially allow for forecasting of hazardous mercury levels to support upcoming deactivation and decommissioning activities.
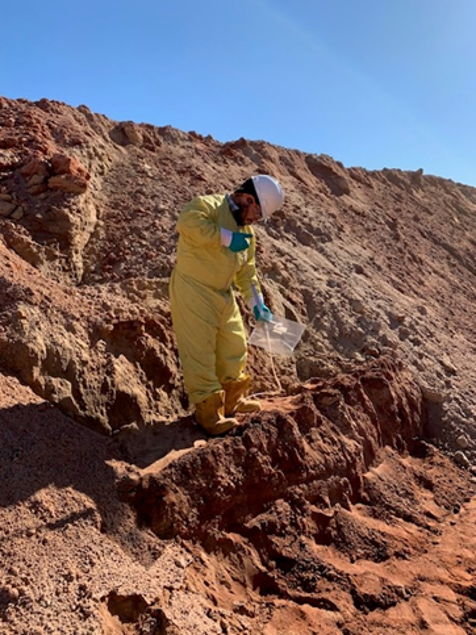
The Moab site is currently developing a Groundwater Compliance Action Plan to address groundwater contamination at the former Moab uranium mill facility. A Groundwater Compliance Action Plan is required by the Uranium Mill Tailings Remedial Action Program and will provide a complete description of planned groundwater compliance activities.
SRNL is currently working with the Moab team to recommend a remedial strategy coupled with an ALTEMIS based long-term monitoring strategy. SRNL will provide site-specific recommendations for implementing this strategy. This strategy includes a mix of the standard sample collection methods combined with installing sensors supported by Artificial Intelligence/Machine Learning models to monitor residual contamination flow into the adjacent Colorado River. This approach, if implemented could significantly reduce monitoring costs while improving the quality of monitoring.
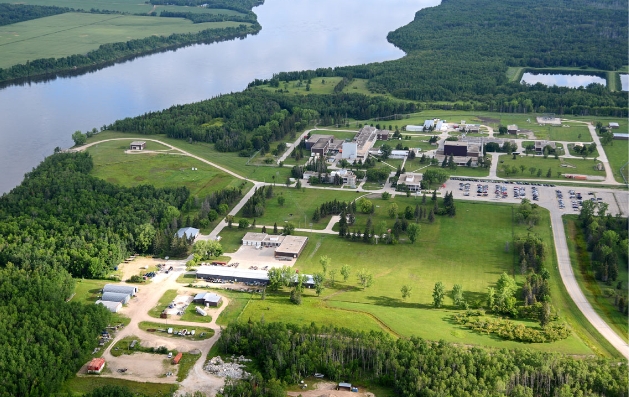
Canadian Nuclear Laboratories has requested SRNL’s support in implementing ALTEMIS technologies and strategies at their Chalk River site in Ontario, Canada. SRNL has traveled to the site and gained an understanding of the site-specific issues. An information exchange is still ongoing and SRNL researchers are formulating a plan to implement ALTEMIS at Canadian Nuclear Laboratories.
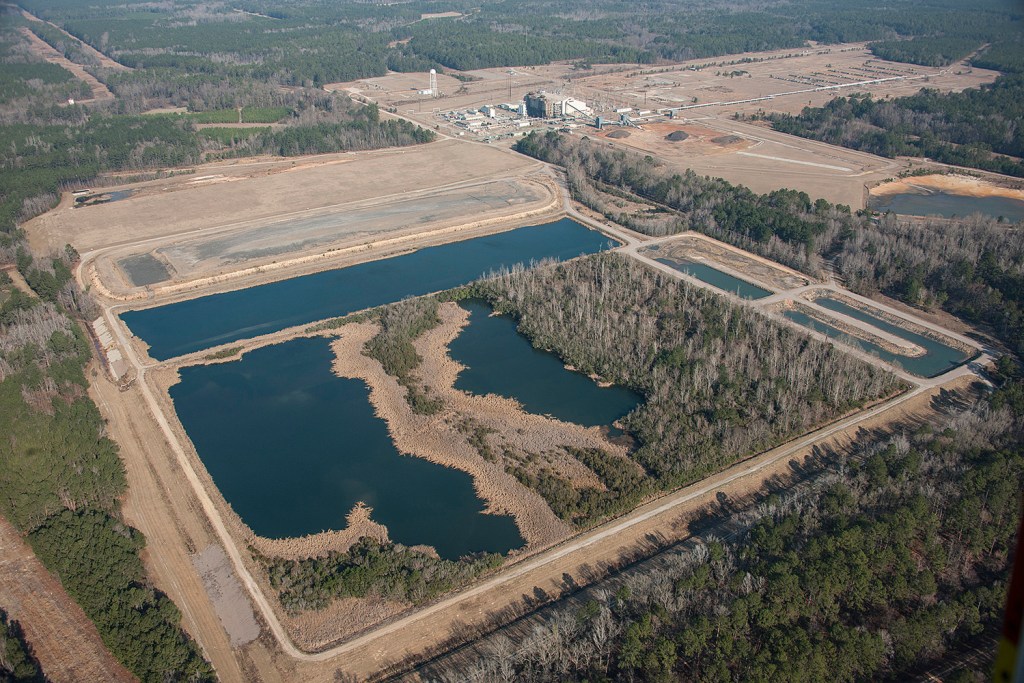
There is broad interest in applying Artificial Intelligence/Machine Learning to sites that have non-radionuclide contaminants, such as those impacted by coal combustion residuals. SRNL has tested the existing ALTEMIS framework at the Savannah River Site D-Area coal ash basin to predict the concentration of heavy metal contaminants, such as arsenic and selenium. Notably, the D-Area datasets contain significantly fewer data points, creating a sparse data problem and an opportunity to continue developing Artificial Intelligence/Machine Learning techniques that are applicable in such cases.